Codon optimization and AI: Tackling a classic synthetic biology problem
Apr 25, 2023
Meet CO-BERTa, Absci’s supervised learning model for better expression level predictions.
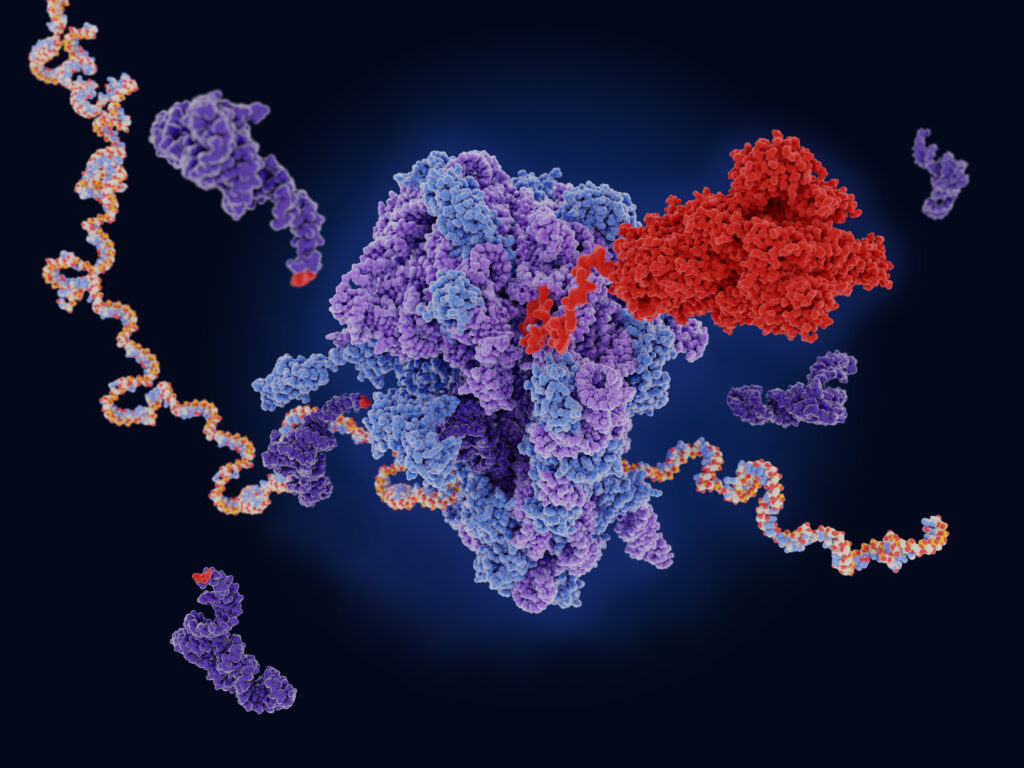
AI has many applications in drug discovery, including target identification, lead optimization, and the structure-function challenge, and AI’s broader impacts are just beginning to be felt in clinical trials, drug manufacturing, and personalized medicine.
One perennial challenge for synthetic biology is codon optimization: how to choose codons to maximize protein production. A recent pre-print manuscript from Absci describes a deep learning-based codon optimization strategy to tackle this common challenge in synthetic biology.
“In any project, whether it’s cell line development or drug discovery, you’re going to need to make protein,” says Rebecca Viazzo, a Senior Research Associate who worked on the wet lab part of the project. “And often, you have to make a lot of protein, especially when you’re screening large libraries. So it’s awesome that we have this new model that can help us in just about any project that we have.”
Here’s the story of CO-BERTa, a deep learning model that combines high-throughput wet lab assay development, data acquisition, and artificial intelligence to optimize expression levels of recombinant proteins like never before.
Why codon optimization matters
First, a little background. Codon optimization is the process of selecting the most optimal codons (the three-letter “words” that specify amino acids) to use in a gene sequence to maximize protein expression.
Why is this important? Well, different organisms use different codons to encode the same amino acids, and some codons are more frequently used than others. This can affect how efficiently a protein is produced in a particular host organism because some organisms preferentially use certain codons over others. By choosing codons that are more commonly used by the host organism, researchers can potentially increase the efficiency and yield of protein production.
In synthetic biology, codon optimization is often used to “recode” genes from one organism to work more efficiently in another. For example, if you wanted to produce a human protein in the lab workhorse E. coli, you might optimize the gene sequence to use codons that are more commonly used in bacteria, rather than the codons that the human gene originally used. This can help increase the yield of the protein and make it easier to produce on a large scale.
Applying AI to codon optimization
With deep learning techniques, researchers can now take codon optimization to a new level by using machine learning algorithms to predict which codons will lead to the highest levels of protein expression. By training these algorithms on large datasets of expression data, Absci researchers have built a model that can predict the expression level of a given gene sequence based on its codon usage. This can help them design gene sequences that are optimized for high expression in a particular host organism, without having to rely on trial and error in the lab.
In creating the model, the Absci team initially attempted a codon optimization strategy by designing coding sequences (CDSs) with sequence profiles similar to the expression host genome. They developed a generative deep learning model called CO-T5, which was trained on CDSs from the Enterobacterales order. The model generates CDSs from protein sequences that are remarkably similar to their natural versions. However, the team found that even though sequences produced from CO-T5 are highly natural, they don’t necessarily express highly.
To address this issue, the team developed a new deep-learning model named CO-BERTa that uses supervised learning to fine-tune the prediction of expression levels based on training data. The team used Absci’s scalable wet lab to generate over 150,000 expression measurements of synonymous mutants from three diverse proteins (to our knowledge, the largest database of its type). After training CO-BERTa on this database, the team found that CO-BERTa can learn and predict the sequence-to-expression relationship for the three proteins in the dataset with high accuracy.
Additionally, CO-BERTa can accurately rank the highest 10% expression variants not seen by the model, demonstrating that the model can generalize to out-of-distribution high-expression examples. The team also found that by training CO-BERTa on multiple protein examples simultaneously, in multi-task mode, accuracy improves compared to models trained on only single protein variants.
To test the generalizability of the model, the team designed high-expression variants of two new proteins, an mCherry and a VHH. CO-BERTa’s designs outperformed other commercial algorithms and demonstrated that the model has learned fundamental rules governing codon optimization.
It fell to Rebecca to organize those final experiments in the wet lab, and there was definitely a kind of “hold your breath” moment when it came to testing the model against the two proteins outside of the training set.
“We spent the better part of a year on this project, building the model and trying it in these new molecules,” Rebecca explained. “It was just a fun project, especially working with such a great and talented team. Science can be difficult — you don’t always see the results you are hoping for. But after months and months of hard work, it was incredibly rewarding to see the codon optimization model we built successfully predict protein expression.”
What this could mean for patients
CO-BERTa is an extremely useful tool for those of us in the lab when we need to increase expression levels of recombinant proteins, including biologics such as antibodies. But what does it mean for the patients we aim to serve?
Put simply, it can help us develop new drugs that are both effective and affordable. By optimizing the gene sequence to use codons that are more commonly used in the host organism, researchers can potentially increase the yield of the protein and make it easier to produce on a large scale. This can lead to a more stable supply of drugs and help ensure that patients receive the life-changing medicine they need.
CO-BERTa highlights the potential of using artificial intelligence in synthetic biology – in this case, to tackle the classic problem of codon optimization. With the CO-BERTa deep learning model, researchers can predict which codons will lead to the highest levels of protein expression, improving efficiency and yield in protein production. It’s another example of how Absci combines AI and synthetic biology on its mission to create better biologics for patients, faster.