Anyone can generate thousands of new AI drug candidates. What matters is one great lead.
Jul 11, 2023
As biotechs rush into AI drug creation, Absci’s Imad Ajjawi helps make sense of the noise.
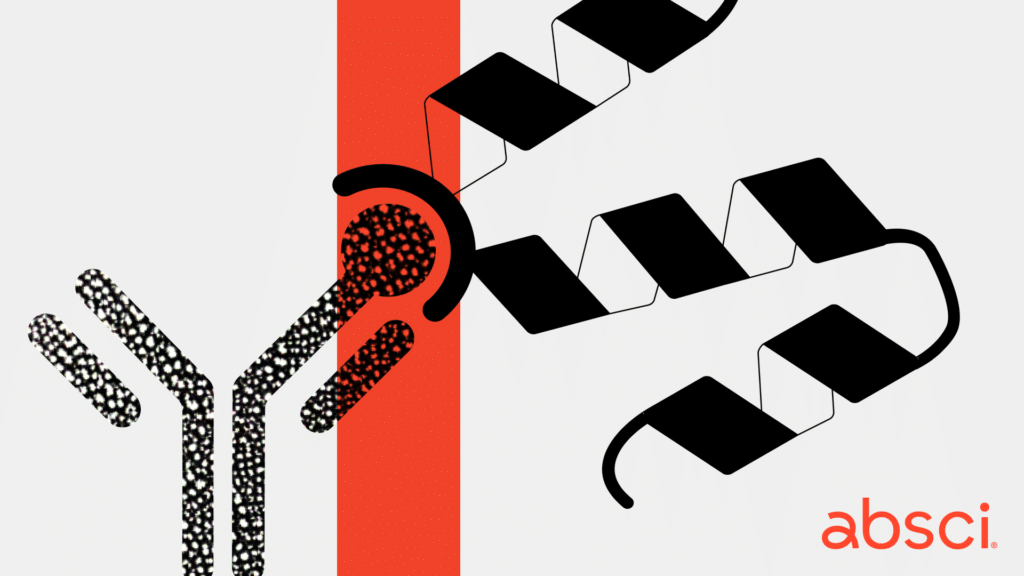
Not long ago, convincing pharma partners about the potential of AI was an uphill battle.
“Five or six years ago when we were pitching AI drug discovery, there were a lot of skeptics,” says Imad Ajjawi, VP of Business Development at Absci. Imad has been talking AI with pharma companies since 2018. That’s when he co-founded Denovium — an AI company that helped pioneer some of the deep learning methods that underpin biopharma today — which was acquired by Absci in January 2021.
Imad still hears some of that skepticism, but the industry has seen what AI can do, and new AI drug companies are popping up every day. Now, pharma is upping the ante.
“Just about anyone can get an antibody for a well-behaved soluble target,” Imad says. “Companies don’t need 10,000 more hits — they need one perfect candidate. And they’re coming to us for help with harder problems: fine-tuning binding affinity for multi-specifics, addressing tough membrane targets, co-optimizing antibodies for multiple drug-like attributes such as affinity, developability and immunogenicity, extending half-life, and even broadening their intellectual property.”
But with as many as 270 companies in AI-driven drug discovery, finding the perfect partner is a lot like finding the perfect lead candidate – like searching for a needle in a haystack. Here’s Imad’s perspective on the growing AI drug discovery industry.
Blazing the AI trail
“We’re in the early days,” Imad says, “and there’s a lot of noise in the market. A lot of people claim to have what we have or have similar offerings, but there are few real case studies to demonstrate success.”
In the pharmaceutical industry, where the failure rate hovers unacceptably around 95%, Imad says potential partners are essentially making a bet. “And to convince a partner to make a bet on Absci, we’ve got to show that we’re substantially better.”
One way to show how well we’re leading the field — and inspire confidence in future partners — is through the manuscripts we’ve made public. Imad points to two key breakthroughs that put Absci at the forefront of the field.
First, Imad says, there was Absci’s lead optimization manuscript showing that its deep contextual language models trained on high-throughput affinity data can quantitatively predict the binding affinity of unseen antibody sequence variants.
“Before that, the state of the art was Sai Reddy’s AI classifier system, which looks at a sequence and tells you whether it binds to a target — yes or no.” Using deep learning, Imad says Absci substantially improved on the state of the art to be able to fine-tune binding affinity. This gives drugmakers a key tool to improve efficacy, reduce side effects, increase specificity, and reduce the dose of a drug.
In a bioRxiv preprint publication, Absci first described the ability to tune binding affinity in trastuzumab, the well-studied HER-2 monoclonal antibody used to treat breast cancer, before generalizing the approach to other targets.
“There’s got to be confidence that we can reproduce that ability for other target-antibody pairs that partners bring to us,” Imad says. “And now we are demonstrating this capability repeatedly – or commercially – for several programs, to the point where we know it’s a reliable AI model and a robust approach that can be applied toward a variety of targets.”
Second, Imad points to Absci’s manuscript showing an industry-first: creating de novo versions of all three heavy chain CDRs. When measured in the lab, the de novo candidates had hit rates 5 to 30 times higher than a random sample from the Observed Antibody Space (OAS).
“We are not just tweaking existing antibodies,” Imad says. “Absci’s zero-shot AI method designs antibodies without using any training data on those specific targets. So we’re getting antibody candidates unlike those found in existing databases.”
“We are hitting a lot of firsts,” says Imad, “which says the platform can deliver as promised, and maybe even go beyond that.”
The biologics bandwagon
Though AI drug discovery is red-hot, the segment is almost exclusively focused on small-molecule drugs. Only a small handful of companies are focused on biologic drugs, which offer greater specificity, enhanced potency, and the ability to target complex, yet-to-be-drugged diseases. In 2022, seven out of ten blockbusters were biologics, making it an extremely attractive therapeutic segment.
“We are seeing more companies jump on the bandwagon,” says Imad, “but Absci was one of the first to focus on biologics, so we’ve got quite a head start compared to others starting now.” He figures that Absci has generated billions upon billions of data points — learnings that have been put back into the algorithms to improve candidate selection.
Imad acknowledges that biologics are intrinsically more complex to design and make than small molecules. But he says that Absci is solving that problem with two technologies that emerged from its decade of experience as a cell line development company: SoluPro® research and ACE™ assay technology.
With SoluPro® research, a heavily engineered E. coli cell line designed specifically to manufacture human proteins, Absci can construct billions of genetically-distinct cells, each containing instructions to make one version of a protein of interest, as well as a different assortment of folding and expression solutions. Then Absci’s ACE™ assay technology evaluates and sorts hundreds of millions of designs to collect the best hits based on target binding, protein quality, and expression titer.
Imad knew that Absci had unlocked biologic drug discovery when Denovium first began partnering with them. “We had about 14-15 different partnerships back then,” he says, “and every time we delivered our AI designs, our partners were slow to get the lab data back for validation and feedback — incredibly slow. Except for Absci — it was one of the few partners that could turn around data fast. It was incredible, and so nice to get that validation quickly. I knew that we could work well together and that Absci was on to something big.”
The next phase of AI drug discovery
Imad believes easy targets are quickly becoming a commodity, and that the immediate future of AI drug discovery probably lies in targets that aren’t quite so simple.
“For the soluble, easy-to-immunize targets in humanized rats and rabbits, there are technologies to do that. You can get antibodies pretty efficiently in about six months,” he says.
Pharma is now turning its attention to harder targets, such as insoluble, membrane-based targets, which represent about 60% of all drug targets. Membrane proteins are particularly interesting because they control so many cellular processes, including signaling, transport, and adhesion.
“That is truly a bottleneck, and that’s where I think our AI can add a lot of value to large pharma and biotech,” Imad says.
And in the future, Imad foresees a time when advances in data, models, and processing enable us to go beyond mere sequence-to-structure and enable us to truly generalize protein-protein interactions. This will one day help take animals and humans out of the drug development process — and drugs will truly be created in a computer and validated in the lab.
“Just like AlphaFold, you can see that AI will learn the protein-protein interaction problem,” says Imad. “It’s not a matter of if, it’s a question of when.”
Until then, Imad is focusing on all the good things we can do today. Like ChatGPT, he says AI drug creation will always be improving.
“It’s true, ChatGPT can get this or that wrong, and you have to check the references. But think about all the other things that AI is already enabling — better diagnostics, semi-autonomous vehicles, amazing artwork with just verbal prompts, assistance writing and automating mundane tasks. The applications are endless, and AI will provide better and better solutions in a fraction of the time. I think our industry understands that, and I can’t wait to see the impact as AI becomes integrated into the drug discovery process.”