Clinical data at the speed of AI: Finding the shortest path to patient impact
Jul 20, 2023
Pediatrician turned pharma innovator Hubert Trรผbel on using AI to translate R&D into patient-centric solutions.
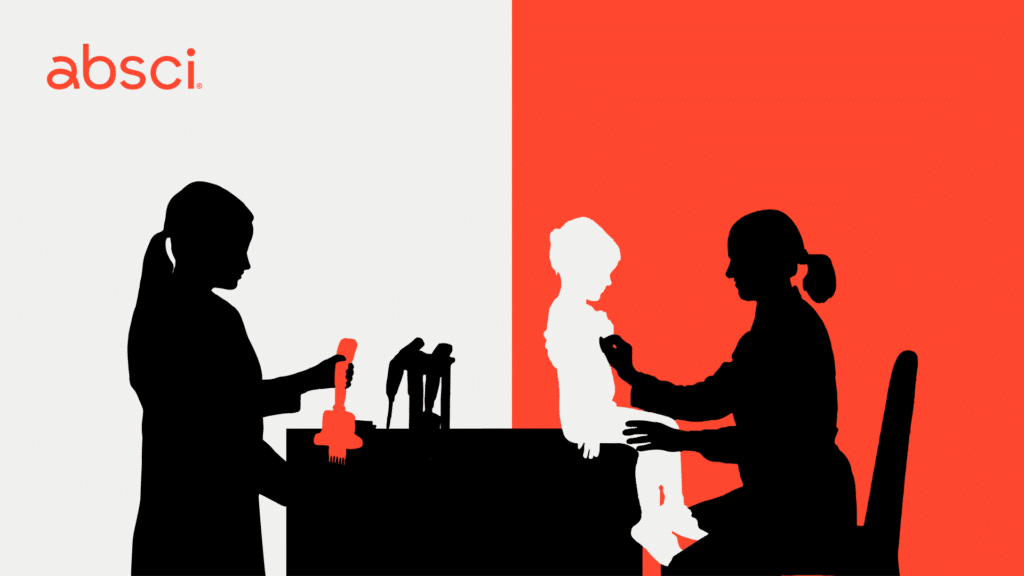
The path from drug discovery to first-in-human therapies is twisty and time-consuming. Prof. Hubert Trรผbel has followed a similarly twisty career path, going from academics to medicine to pharma. That experience has contributed to his success in bringing impactful drugs to patients, and it colors how he balances an openness to new technical and professional frontiers with a disciplined eye toward clinical results.
โI have had a long and convoluted career in the pharma sector as well as in the academic world, all driven by chance,โ he says. โAnd so my success factor, I think, is giving chance an opportunity to strike.โ
Today, as Chief Medical Officer of AiCuris and a member of Absciโs Scientific Advisory Board, Hubert is less interested in wandering than in finding the most direct path from research to patient impact. He sat down to discuss with us what is top-of-mind for him these days: the shortest path to clinical data, the true meaning of translational success, and AIโs potential for patients with rare diseases.
A convoluted path to drug discovery
Hubertโs interest in translational medicine emerged during his pediatrics residency in Germany when an opportunity came to join a biotech startup. He left his residency and took the plunge, but the company failed just 18 months later. He returned to complete his residency but kept coming up with ideas that couldnโt be pursued in academia.
โI did a fellowship in the US and came back with even more research ideas,โ he says. He met some folks at Bayer, and before long he found himself having coffee on Saturday morning at the home of Andreas Busch โ then a Bayer executive and Absciโs current Chief Innovation Officer.
He began as a self-described โlab ratโ but quickly rose through the ranks as lab head, department head, and experimental medicine lead, all by โfollowing projects along the value chain and translating them into the clinic.โ He ultimately led all of Bayer’s clinical research development before moving back to biotech to serve as Chief Medical Officer at AiCuris to help develop anti-infectives.
The shortest path to clinical data
Dr. Trรผbel brings his translational perspective to Absciโs Scientific Advisory Board, where he feels heโs โin the front seat of antibody development innovation.โ His main focus there is on using AI innovations ike designing HCDRs from scratch and multiparametric lead optimization to go from preclinical research to high-value clinical data in the fastest, most direct way possible.
โItโs a long and daunting process at times,โ he says, with experimental, manufacturing, and regulatory challenges all along the way. โWhat made me successful at Bayer, and what I bring to Absci, is thinking about the most efficient way to get to a clinical endpoint to help validate a project. You need the data to show a project is justified to be in your pipeline,โ he says.
Hubert loves coming up with ways to validate a target product profile, or TPP. The TPP is the starting point for any new drug. It describes the indications, efficacy, safety, dosing, and delivery of what youโre trying to make. The drug design process is complex and has to be data-driven, he says. But above all else, it must center on patient needs if it hopes to become a blockbuster hit.
โIt all starts with an unmet need,โ he says. โThatโs what drives innovation, whether itโs a drug or anything else you want to bring to market.โ
Reverse-engineering the patient experience
In developing the ideal product at the center of the TPP, drugmakers also need to account for other products in the space.
โLet’s talk about PK,โ says Hubert. โImagine thereโs another drug on the market for your disease target, and patients take it twice daily. To compete in the market, your drug might need to be once daily.โ This, he says, is the basis for what data-driven attributes you need to achieve in your pharmacokinetics โ how to keep the active pharmaceutical ingredient in the bloodstream over a period of time, for example. Whatever the PK attribute, the TPP helps you reverse engineer the drug you actually want.
Once you define a drug that meets the needs of patients, physicians, and regulators, โthen itโs a matter of going through the hoops,โ he says. โYou will need to validate different aspects of your target product profile, and today a lot of this validation can be done in silico.โ
Translational success in AI drug discovery
Going in silico is key, Hubert says, because the greatest driver of translational success is time saved in achieving a successful clinical data point.
โWhen you look at drug development today, a lot of time is spent on certain steps in the analysis,โ he says. Examples include finding the right antibody-target match or running a dozen assays on millions of compounds. Such processes are extraordinarily expensive, slow, and labor-intensive. And when you finally choose a lead candidate, you then have to optimize it in vitro and in vivo. Hubert believes that a lot of the data needed to validate and optimize candidates is already out there, waiting to be put to use in machine learning models.
โAI will allow us to move faster through these stages,โ he says, in a sense enabling drugmakers to jump through more of those hoops with the click of a button. And in terms of peak sales and market exclusivity, Hubert says that the time savings represents enormous value for pharma.
The patient opportunity: Rare diseases and beyond
But the greatest opportunity in AI drug discovery lies in bringing life-changing medicines to patients.
Hubert believes that rare disease communities will be among the first to benefit. Today, drug development for rare diseases depends on the same kinds of animal models and human studies used for other diseases. But this presents a double challenge for rare diseases. First, there are few animal models for rare diseases. And second, itโs often difficult to find enough rare disease patients to conduct a human clinical trial.
Hubert expects that the shift to in silico approaches will ultimately enable AI drugmakers to do more drug development outside of animal models and human studies. He says these technical advances are coming just as regulators are giving drugmakers new leeway in finding treatments for the 6000+ rare diseases impacting humans worldwide.
And rare disease communities will not be the only winners. Whether itโs speeding the discovery process, increasing clinical success, or making more personalized therapies more economically viable, the same AI advances that help rare disease will also advance drug development broadly.
As Hubert puts it: โIf AI can shorten the development and resource needs, thatโs good for all patients.โ