Beyond the hype: What pharma really has to gain from de novo antibodies
Feb 02, 2023
Pharma veteran Dr. Andreas Busch discusses what drugmakers can reasonably hope to expect from AI drug design in the near-term.
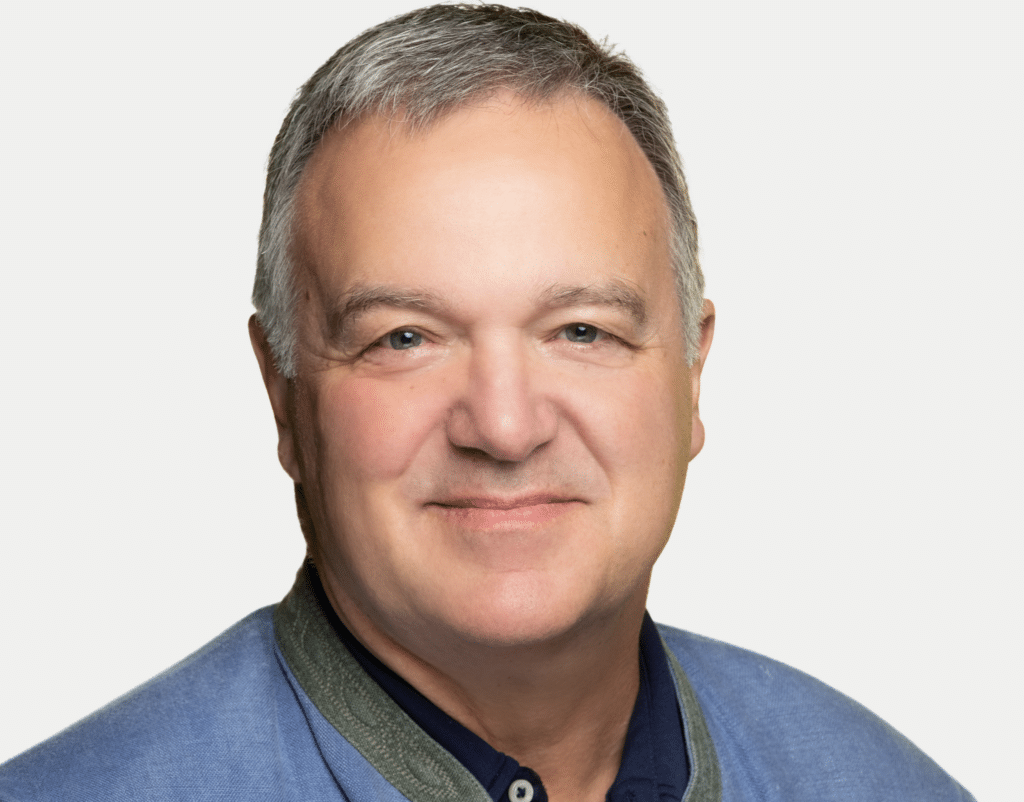
For someone with so much success bringing drugs to market, Andreas Busch comes across as quite the realist.
โWe should always remind ourselves what weโre actually affecting versus what weโre doing with the technology,โ he says. โYou can produce the best antibody against pretty much every epitope imaginable โ high affinity, high solubility, high Naturalness, whatever pH you need, you name it โ itโs going to be a high-quality antibody. But if your target is off, it’s worth nothing.โ
And in case you needed more reality check, Andreas likes to point out that in addition to these scientific challenges there isnโt a blockbuster out there that hasnโt been halted numerous times during its development.
โDrugs get delayed and often put on the shelf for strategic reasons that have nothing to do with their quality. Projects are put on hold because of funding, or perhaps the strategy officer changes. This can obviously play a huge role in the success and speed at which new drugs come to market,โ he says.
So, is AI going to fix these problems? No. But Andreas believes AI is going to have a significant impact on biologic drug discovery. Not just in areas that Absci focuses on โ antibody design and target discovery โ but also in safety, toxicology, and more.
โWith present technologies, we can get pretty decent antibodies,โ he concedes. โThere are many good antibodies on the market already that didnโt use AI. But the value in using generative AI to create de novo antibodies is that we are much faster in optimizing antibodies across multiple parameters.โ And that time advantage is huge โ especially at the beginning and end of a drugโs life cycle.
In drug discovery, time is more valuable than ever
With zero-shot generative AI, Absci thinks it can reduce the time from target identification to clinic by about two years. What’s the consequence of those two years?
โThe first is simple to understand,โ says Andreas. โIf you commercialize your drug two years early, that gives you two more years of sales at the end of your patent life. If you’re talking about a billion-dollar-a-year drug, that could mean $2 billion in revenue at the end of the life cycle. That’s very simple. Generative AI can also provide new opportunities around life cycle management of the drug post-patent cliff.โ
The second advantage may be even more important to appreciate, he says, and it pertains to todayโs highly competitive drug discovery environment.
โIn years past, you often knew whether you had a leadership position on identifying a certain signaling pathway or target,โ he says. โToday, whatever target youโre working on, you have to assume that five other companies have also discovered it and are working on it, too. In such cases, a month or two could make the difference between bringing your blockbuster to market and being shut out by a competitorโs patent.โ
He adds: โIf you have a chance of being two years faster, that puts you in a completely different competitive situation.โ
If mice could talk
In addition to the time advantage, Andreas believes that AI can just do a better job of optimizing antibodies across multiple parameters than conventional approaches.
โIf you do a mouse campaign to identify an antibody, you canโt tell a mouse, โWe donโt want just any antibody from you โ it has to be highly soluble, have the right binding, the right pH dependence, and so on.โโ Andreas says you can get high quality antibodies, but itโs going to take years to get all the characteristics you want.
โWith predictive AI approaches, we can optimize antibodies to have many specific parameters all at once.โ
โAnd if our predictions around what we call a Naturalness score are correct, then we can predict things like immunogenicity, which is of huge importance to pharma โ making sure you don’t form autoantibodies or any immunogenic reactions. Thatโs a huge value to the drugmaker.โ
IP opportunities through AI
Andreas believes that because generative AI can also provide numerous highly diverse sequences that bind the same epitope, a drugmakerโs IP estate has the potential to be meaningfully expanded.
โThe zero-shot AI approach is a method that specifically removes any training data for the specific targets of interest,โ Andreas explains. โSo weโre teaching it the general principles of good antibody design, but weโre not biasing it toward known antibody sequences in existing libraries.โ
Andreas says that this approach generates designs that are quite diverse compared to known antibodies, giving drug makers greater opportunities with regard to patent estate strategy.
In our recent bioRxiv manuscript, we showed how we can use zero-shot generative AI to design HCDR3 regions for trastuzumab with extreme edit distances and higher affinities.
โWhen we do talk to partners about patent opportunities, they understand the potential value really well.โ
Creating drugs at the speed of AI
The field of AI drug discovery is moving fast and changing the way drugmakers think about technology partnerships, addressable markets, and the drug discovery process itself. Itโs moving so fast, in fact, that Andreas believes weโre shifting from a paradigm of drug discovery to one of drug creation.
โFirst serving on Absciโs Board, and now as Chief Innovation Officer, Iโm more convinced than ever that Absci is dismantling the paradigm of drug discovery with generative AI,โ says Andreas, โallowing us to achieve our vision to go from discovering or finding drugs within existing libraries to creating drugs in silico on a computer.โ